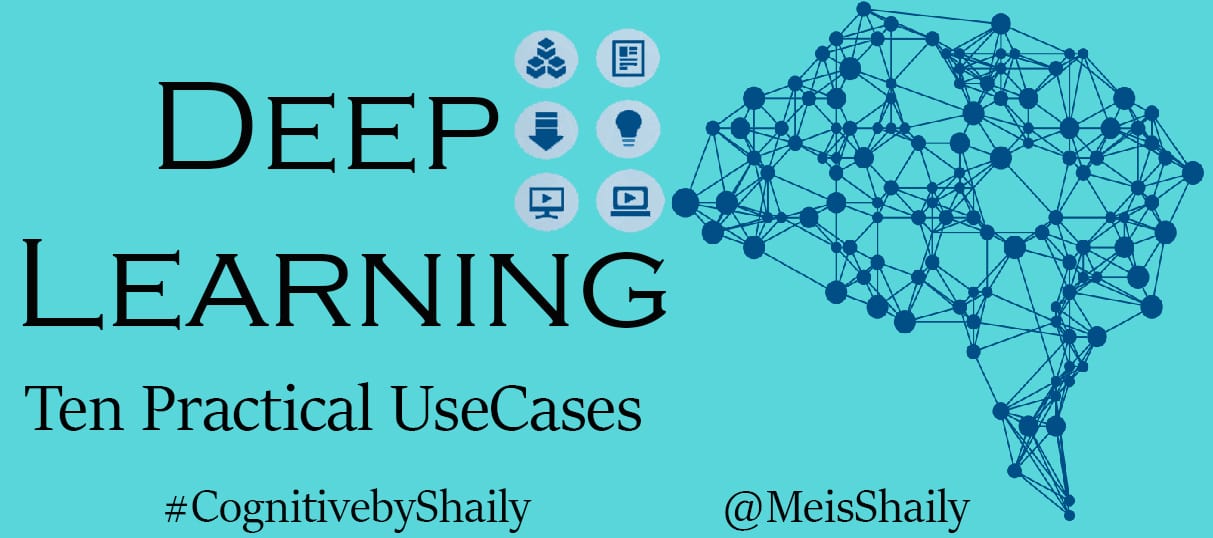
Ten Practical DeepLearning UseCases
If you have been wondering how there is always so much to do yet so little time, time has come when you can finally put a halt to that thought as artificial intelligence has just the things you need. In fact, with artificial intelligence and cognitive computing you can get things done with greater efficiency and much lesser effort than you thought was possible. Deep learning, which is one of the technologically superior methods behind the formulation of Artificial Intelligence, traces the evolution path of human intelligence design to develop machines that can perform tasks on their own and without human supervision helping in automation.
It is very interesting to note how deep learning has altered the way we operate in various aspects of daily activities and necessity areas. Let us take into consideration 10 practical use cases of Deep Learning Techniques that have been witnessed in the last few years.
ONE: Facial Recognition using visual intelligence of machines
It is astounding how security cameras have grown over the years. Today, machine learned security cameras used at airports or secured parking lots use their analytical ‘mind’ to not only record a particular intrusion but also review and analyze the scene.
Objective: To identify, detect and track persons of interest, parked vehicles, missing luggage and many more using CCTVs or other high-end cameras.
Deep Learning Technique: A VPU or a Vision Processing Unit, is a much advanced deep learning product that takes over the conventional CPU or GPU. A lot of processing and machine learning is conducted on the device which helps it to analyze a particular scene. It uses Convolutional Deep Learning Models to detect and track objects and individuals.
Inference: Most of the airports are now able to use the deep learning techniques to identify and track persons of interest (e.g. terror suspects, etc.), track your luggage and detect any suspicious item very quickly. These VPU-enabled security cameras installed at airports, generate alerts the moment they find someone leaving their luggage, thus making it possible to detect airport security threats within minutes. Installed in traffic scenarios, they can also recognize driver-less cars and help them find the right parking spots.
TWO: Understanding customer behaviour in ecommerce
Deep learning is being used to develop techniques to get clearer ideas about customer wants and expectations in the ecommerce industry. Let us see how.
Objective : To understand customer behaviour and their propensity to purchase a particular product.
Deep Learning technique: Recurrent Neural Networks (RNN) has a potential for higher accuracy than any other machine learning technique. An RNN network is generally made up of computational cells which are fed with consumer histories. These cells are provided with a given-time step, which helps the program to understand the consumer’s step-wise behaviour when he/she is viewing a particular product on a site. For example, an RNN cell will note when the consumer clicks on an ad, is directed to a site, views a product and adds it to the shopping cart. These RNNs are often long-time memory cells and they remember and relate customer behaviour across a line of products.
Inference: E-commerce sites, such as ebay and Amazon, are greatly benefited through Deep Learning. The entire session journey of consumers are noted. The longer and dynamic an event is, the greater is the propensity of people to click on the ‘buy now’ button. Once a particular consumer behaviour is noted in case of a range of products, the site is optimized to ensure that the next time a similar consumer visits, they get a more engaging experience so as to convert into a purchase within the shortest time.
THREE: Having a private tech self-support
With the introduction of computers, there were recurring talks about how machines would replace humans one day. It is now possible to minimise human-labour and improve efficiency with machine learning.
Objective: To improve work efficiency of an organization by installing machine-learned support staff unit, instead of hiring manual labour.
Deep Learning technique : Algorithmic Scheduling Agents are built using a blend of deep learning algorithms that ensure distribution of labour so that a number of tasks are performed within the shortest possible time and at minimum costs. Agents like cyborg systems are developing using the technique.
Inference: For calendar coordination and scheduling, we have Clara and to gather staff report and consolidate meeting information we have Howdy. Google Now is the preferred program for keeping on schedule through proactive alerts, and for follow-ups after meetings, GridSpace Sift is a brilliant manager.
FOUR: Transforming industrial sector through Internet of Things Data
Industrial Internet of Things (IIoT) refers to the interlinking of physical devices, buildings, vehicles and other items through electronic, sensors and software so that the data is collected and exchanged.
Objective : To initiate preventive maintenance of machine units through machine learned sensors, in order to improve longevity and improve performance.
Deep Learning technique: Sensor data analysis. By using machine-learned sensors and IIoT, accurate maintenance time predictions are made. Timely maintenance of machines is very important to ensure that work for a long period of time. Initially manufacturers would mostly rely on guesswork to time the maintenance interval of machines. IIoT is combined with predictive analysis gives the perfect maintenance times. Machine learning of prior downtime incidents also play a great role.
Inference: In several countries, chemical manufacturing units and aircrafts units are installing machine learned sensors to get complete idea of new challenges and insights. This has helped in reducing pitfalls and machine breakdown, thus considerably reducing costs and maintaining a healthy and hygienic environment in manufacturing units.
FIVE: Convolutional neural networks finding minerals
Multi-special satellite images have helped found mineral resources in several countries like Australia. Geologists have been able to use the mineral indexes integrated with the algorithms to find traces of minerals (even gold) deep inside the earth’s crust.
Objective: Detecting mineral deposits using multi-special satellite images have helped found mineral resources.
Deep Learning technique: Transfer learning is a great way for convolutional neural networks to carry out this function. These networks are pre-trained and fed with data over minerals and geographical location accuracy, before they are operated. Converting information into geospatial data is the challenge that has to be undertaken.
Inference: Geologists in Austria, are currently using the procedure to locate minerals in relatively unexplored areas of the land.
SIX: Deep learning facilitating automatic grading of eye diseases
GPU-based medical research machines are now using deep learning methods for medical break-throughs.
Objective: To simplify the processes leading to eye disease detection, providing the researchers guidance based on historical information.
Deep Learning technique: Convolutional Neural Networks are being used to put diabetic retinopathy diseases in various categories. The devices are machine learned to analyze stages of the diseases, to ensure that diagnosis offered is accurate. Effectively programmed web-based systems comprising of 1000s of eye images are used to help the machine system analyze and identify the right stages in the diseases, making way for accuracy.
Inference: Researchers today are very close to developing digital assistance to radiologists, ophthalmologists and other clinicians. Significant progress has been made in the field in the past years.
SEVEN: Defining personal style through artificial intelligence
Objective: To provide fashion brands a clue about customer tastes and the fashion style that will attract them.
Deep Learning technique: Fashionistas visiting sites of top clothing and accessory brands give the machine-learned sites an idea about colour, textures and styles preferred in a particular season or activity. This includes telling them the size, colour and pattern of clothes and jewellery that would suit them the best. Work is on, over such a neural network induced software technology are helping help stylists and fashion industry professionals to make their brands lucrative for buyers. Online retail stores are using this technology to cater to the individual requirements of their customers.
Inference: Visit a page of your favourite shopping site a few times and you will find that products as per your preference is displayed on your personalized page. Thus, you work of choosing and shopping has been made simpler.
EIGHT: Deep learning reducing error rate for diagnosis of breast cancer
The massive research work that is being done over breast cancer diagnosis, has create a colossal knowledge base for scientists from where information assortment is often a problem.
Objective: To use the compact data for enabling quick and error-free diagnosis.
Deep Learning technique in use: The technique used, involves a great role play by cuDNN-accelerated deep learning framework. The computational models were fed with enough data that has been researched so far, making the machines active support for pathologists. Tumor probability heatmaps are used to find the probability of a certain patch containing cancer.
Inference: Adoption of this machine-learning method has enabled pathologists working with computers, outperform independently working pathologists Machine learning has been adopted by several breast cancer research organizations today. The algorithms put together in the technologically enhanced machines, help pathologists identify cancer cells through lymph node images.
NINE: Deep learning is now a part of baby monitor systems
Advanced baby monitors are growing in popularity because of their learned ways to watch a baby. Let’s see how.
Objective: To develop a system which helps parents to keep an eye on babies from a distance and alert them before the baby falls on the floor.
Deep Learning technique: DIGITS or Deep Learning GPU Training System fused with Caffe deep learning. This methodology is used for upgrading conventional baby monitor models. By feeding hundreds of images and information about baby movements and postures, parents can always have an idea about the right and wrong of the child, through timely alerts.
Inference: Instead of conventional baby monitors that just track breathing and heart-rate of children, advanced baby monitors have hit the market that detect wrong postures and movements of the baby, generating alerts to parents from time to time. Thus, if you baby is accidentally sleeping on her stomach, momma can get an alert in the kitchen through the baby monitor and rush to her cot to perfect the posture. Furthermore, if a baby is sleeping on the edge of the bed, an alert is sent to the mom, so she can settle the baby before the baby falls.
TEN: Machine learned cars can now find their way through complex traffic routes
It has always been believed that robots are great when it comes to carry out a task fed into them, but cannot logically reason their way through a problem. Deep learning is all set to change that.
Objective: To create a system that understands connections between a multiple sets of data.
Deep Learning technique: Differentiable Neural Computer (DNC). This method combines deep learning with an external memory that can relate complex sets of data and draw connections to logically reason out things, without human supervision. Pattern recognition technique creating linkages between various events within the data points.
Inference: DNC has been developed by Google’s DeepMind and today it is being installed within cars so that if it is simply presented with maps, lines and stops, it can take the shortest and simplest route to navigate from one point to another. Thus, making it possible for new drivers to make through new roads without difficulty.
From the above mentioned 10 scopes, we can surely conclude that machine learning through neural networks is picking up speed with their increase in popularity all over the globe. Continuous researches and improvisations in the field can give us many more scopes and global fields where deep learning can ease human involvement and accelerate productivity rate.
You can read more examples in my next article on Deep Learning:
Practical UseCases of Deep Learning Techniques… Part II