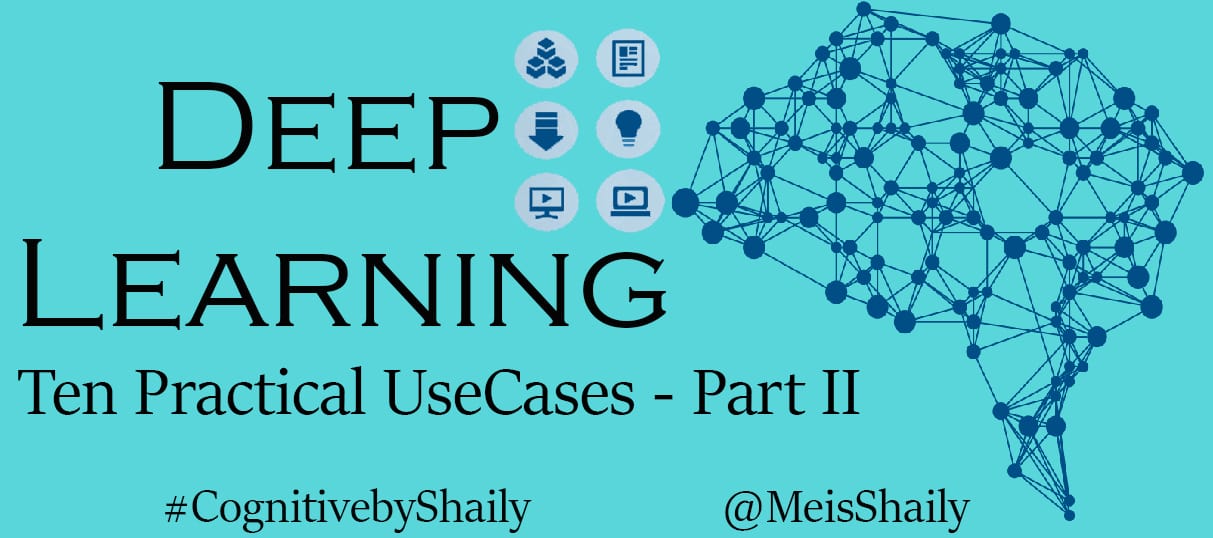
Deep Learning Practical UseCases – Part II
The enormous and raging wave of change that has hit our world in the last decade, has got some of us thinking and others reveling in their glory. The internet and evolving technological practices have increased possibilities. Man and machine collaboration has got us introduced to automated virtual work and communication systems everywhere in the world. Deep Learning has given birth to several real-life applications that have lessened human control and involvement in several spheres of life. The result? Quicker and more efficient completion of work in daily lives with greater accuracy and perfection in research work.
The immense popularity of the Practical Deep Learning UseCases blog was enough encouragement to look at more such UseCases.
ONE: Voice Activated Search Mechanism
Smart phones have surely made our lives easy. But, with minutes slipping away from between our fingers, we have got to size down the length of an operation to achieve maximum efficiency within the shortest time.
Objective: To bring down the time involved in smartphone search processes by enabling the machine to process commands through natural language voice inputs instead of typing.
Deep Learning technique in use: NLP (Natural Language Processing) machine learning which is an updated approach to psychotherapy and personal communication, is the one to go for. This is used to make media files as well as content available on the web searchable to ensure vocabulary augmentation of the platform.
Outcome: Instead of typing on the search tab, one can now simply speak words or phrases on the phone. The underlying technology processes the spoken words and helps the user to access exactly what he has asked for. A similar technology can also be found in Xbox versions. A revolutionary name in the block is that of Azure Media Indexer introduced by Microsoft that enables users to index video content based on everything that has been spoken in the video.
TWO: Data sensors on players making the NFL a bigger sensation
The craze associated with soccer is notched up a level, with fans of the National Football League getting treated with detailed data about the game through the utilization of Big Data in the soccer industry.
Objective: To give football fans a detailed statistical analysis of player form, speed and position through sensor pads installed in the costumes of the team.
Deep Learning technique in use: Data sensors built on convolutional neural networks used to identify and track objects and tags attached to them through electromagnetic fields. These data sensors installed in the costumes of the players help in data collection and analytical assessment.
Outcome: NFL is currently working with a tech-firm Zebra to carry out the job of data sensor installation and statistical analysis. With a chief information officer now hired within the business, individual teams can form their own applications to harness the data to improve team performance during practice hours. NFL teams can therefore reap the rewards of machine learning.
THREE: Deep learning in drug discovery
There is always a need to develop new drugs to cater to the requirements of modern medical practices. But drug-development is highly expensive and a very time-consuming process. Deep learning super computers are thus helping to predict the effect of successful drugs through cutting-edge chemical modelling algorithm.
Objective: To shorten the process of drug discovery, by using deep-learning algorithms on supercomputers.
Deep Learning technique in use: Computational toxicology programs devised using deep neural networks.
Outcome: TwoXar founded by two men in Palo Alto took the help of tech investor Andreessen Horowitz and their DUMA™ Drug Discovery platform. By using large public data sets containing information that has been collected over decades of research work, the platform was able to accurately predict the success rate of several drugs. A conclusion that could have taken decades to reach.
FOUR: Adding sound to silent movies
Most people happen to shoot silent videos of tutorials or simply daily life events. Sound mixing and adding background score might not be their forte. And now, it is not even necessary since deep learning neural networks can do the same with greater efficiency.
Objective: To incorporate appropriate background sounds within videos, without human involvement.
Deep Learning technique in use: Both convolution neural network and LSTM (Long short-term memory) recurrent neural networks are used for this. The LSTM network is perfectly suited to learn from experience and can classify, predict and process time series.
Real world application: Students of the Massachusetts Institute of Technology devised a sound system that simply watches a “silent video clip” and generates accurate sounds to go with it. Termed as the Visually Indicated Sound System, the system was trained to analyze sounds when sticks, prods or scrapes were hit on rough or even liquid surfaces.
FIVE: Image-based question answering
Predicting answers to simple questions might be a difficult task for a human mind that is occupied in thousand different areas, but not for a machine.
Objective: To make machines used in defense and airport security more competent in image recognition and data analysis from them.
Deep Learning technique in use: Deep Learning techniques like RNNs and visual semantic embedding are used. This helps machines relate similar patterns, lines and colors to images and what they denote.
Outcome: Several research organizations like the Pohang University of Science and Technology, Korea are now conducting detailed research in the field. Such organizations are lending these virtual systems fed with QA data sets to the top organizations in the world to help them avoid difficult evaluation issues.
SIX: Deep learning for cyber security
With hackers trying to infiltrate even the most difficult virtual system, application of deep learning methodologies can help cyber security companies predict where the next attack may come from.
Objective: To offer better cyber security for safe and secure online operations
Deep Learning technique in use: GPU-powered advanced neural network schemes are at work to build an advanced security system. Convolutional neural networks are at work here. Deep learning’s capability of identifying malware instinctively result in comprehensive protection on any device, platform, and operating system.
Outcome: Deep Instinct, a very promising Israel and San Francisco based company, is offering such high powered neural network scheme for cyber security which is able to predict the next cyber attack before it happens. Threats are identified and blocked in real-time before any harm can occur. Protection focuses on prevention instead of on post-attack crisis management. As a result, zero-day threats and APT attacks are immediately detected with unmatched accuracy and prevented.
SEVEN: Aerospace drone technology powered by deep learning techniques
The United States government though has been supportive of drone flights, they have also put strict restrictions to limit their indiscriminate use. Drones are generally used for photo journalism, search-and-rescue, agriculture and several other such places. But, in densely populated or undulated regions it is difficult to carry out certain operations.
Objective: Help drones operate with ease in rough terrains, skies and even unclean weather, and without road maps using advanced techniques.
Deep Learning technique in use: Use of single neural network along with edge detection techniques, is helping in making drones independent and pro-active. This way drones are able to access and understand terrains and conduct safer operations without causing accidents.
Outcome: Today Swiss researchers in the University of Zurich and NCCR Robotics have been able to train drones to understand hiking trails through a lot of images. At the end of the study, the drones were able to reach up to a point and recognize the same with 85% accuracy.
EIGHT: Digital Marketing getting a new boost with deep learning
Digital marketing and SEO is all about reaching out to the right client base through the virtual portal and deep learning is helping several companies in achieving exactly that.
Objective: To use the most updated procedures to help companies get prospective clients within the shortest period of time through digital media.
Deep Learning technique in use: By using machine-learned Artificial Intelligence Systems and deep learning neural networks like RNN, companies like Google are able to bring proper search queries to users all over the world.
Outcome: A premier digital marketing company Salesforce acquired the deep learning company Metamind to personalize customer support. Along with other functions, Metamind analyzes signals from certain sources like social profiles to give proper idea about the quality of a lead. This way, it has facilitated several companies in the last few years, to get to the first page of Google and other popular search engines, once a search query comes up.
NINE: Deep learning in logistics
In several countries, robots are being used in warehouses for picking and packing stuff. These robots can become better at their job, if they are trained with deep learning techniques.
Objective: To use robots to conduct different packaging tasks by giving them the learning of hard and delicate objects and the handling requirement of each.
Deep Learning technique in use: Use of RNN powered sensors and other upgraded algorithms within the robots, help them differentiate between objects.
Outcome: A Robotics Institute in Netherlands have recently come into news as the proud winner of a robotics competition where their deep learned robots emerged first in picking items and finishing a whole process, faster than most Cognitive systems in the world. Thus, these robots can replace human involvement in mundane and time-consuming packaging tasks. Hence, Cognitive Systems can be used in several spheres to minimize human involvement and improve efficiency.
TEN: Cognitive Systems detecting correct weather patterns
A lot of events in the business, television, sports, food and other industries are dependent on weather patterns. Today, several government organizations are upping their detection systems with deep learning upgrades for accurate weather reports.
Objective: To train systems and enable them to recognize extreme weather events based on the data available in visual pattern recognition.
Deep Learning technique in use: A deep convolutional multi-layer neural network is the method in use.
Outcome: IBM is currently focusing on helping wind and solar companies to initiate better weather prediction and accelerate the rate of alternate energy production. The company has managed to offer a 30% improvement in accuracy rate of their weather models, through the development of a world-class weather analysis technology based on deep learning.
There are many more deep learning techniques which are being used in our day to day life without we knowing much about it. Cognition is changing our world and deep learning is driving most of it.